Businesses in the retail, banking, and logistics sectors often have complex analysis needs, such as semi-cumulative calculations, many-to-many relationships, and time-window analysis. When SQL is dealing with these scenarios, things can get complicated. Is there another solution to deal with these types of problems? The answer is yes: MDX.
This article will introduce the differences between MDX and SQL starting with some basic concepts, BI semantic models, and analysis scenarios. Keep reading to learn why MDX can often be more suitable for complex analysis scenarios than SQL.
MDX and SQL Basic Concepts
What is MDX?
MDX (Multidimensional expressions) is a query language for OLAP cubes. It was first popularized by Microsoft in 1997 as part of the OLEDB for OLAP specification and later integrated in SQL Server Analysis Services (SSAS). Since then, it has been widely adopted by OLAP database vendors.
Examples of MDX query syntax are as follows:
- SELECT <Axis Expr>[ ,<Axis Expr>]
- FROM [cube]
- WHERE <set>
What is SQL?
SQL (Structured Query Language) is a programming language for defining, adding, retrieving, and managing data from relational databases. The SQL specification provides a wealth of commands and capabilities:
- Data Query Language (DQL) for querying data
- Data Manipulation Language (DML) for addition, deletion, and modification of data
- Data Definition Language (DDL) for defining, modifying, and metadata management
- Data Control Language (DCL) to establish authority, transaction rules, and access control
In order to explain the difference between SQL and MDX, this article will only go over the query part of SQL. Examples of SQL query syntax are as follows:
- SELECT <column expr>[, <column expr>]
- FROM [table]
- WHERE <expr>
What are the main differences between MDX and SQL queries?
The main body selected by MDX, namely the SELECT part, is a dimension measure or its expression. The body of SQL selection is the column or columnar expression.
The body of the MDX query, the FROM part, is querying from an OLAP cube, data that is pre-joined and aggregated. The MDX query does not need to specify the join relationship. The main body of the SQL query is a relational table, which is a detailed record. When querying with SQL, you need to specify the join relationship between the tables.
MDX and SQL Syntax
MDX can be equivalent to SQL in many cases, such as the below query, the sales of electronic products in 2019.
Expressed in MDX as:
- SELECT [Region].[Province].members
- FROM [Sales]
- WHERE ([Time].[Year].[2019], [Product].[Category].[Electronic Product])
Expressed in SQL as:
- SELECT region.province FROM sales
- JOIN region ON sales.region_id = region.id
- JOIN time ON sales.time_id = time.id
- JOIN product ON sales.product_id = product.id
- WHERE time.year = 2019 AND product.category = "Electronic Product"
BI Semantic Model
Currently, mainstream BI products (Tableau, Power BI, Qlik, etc.) support connecting to relational databases through an SQL interface (JDBC/ODBC). They support connecting to multidimensional databases through an MDX interface (XMLA). However, the semantic models obtained by traditional BI tools through the two interfaces are quite different.
The MDX semantic model includes dimensions, measures, hierarchical structure, etc., without the need for analysts to define business semantics on the BI side. This has the advantage that data engineers and modelers can uniformly define business rules in OLAP tools. With MDX, business users don’t need to understand the complexities of the underlying data structures to conduct analyses. They can directly use the dimensions, metrics, hierarchical structures, and calculated metrics synchronized to the BI tools.
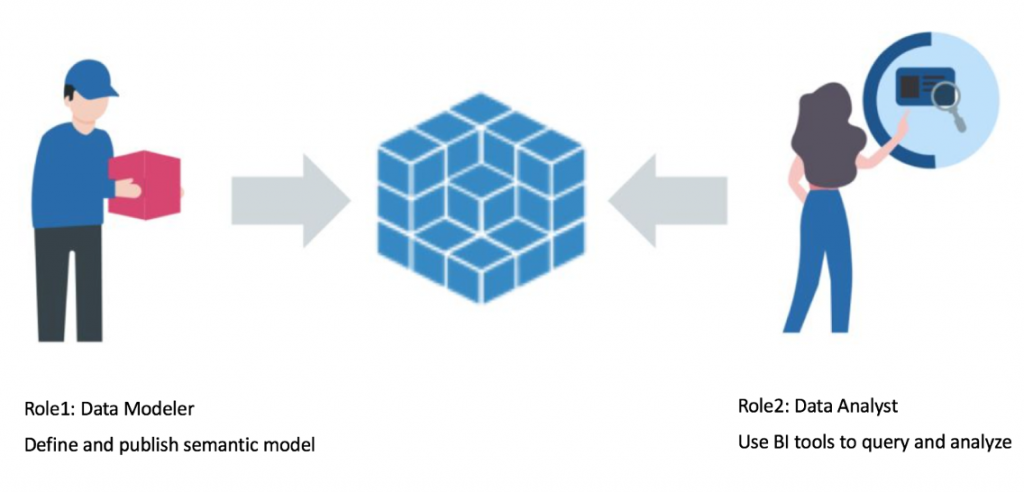
In addition, MDX has stronger capabilities for complex analysis scenarios than SQL. For complex scenarios such as semi-additive, time window analysis, many-to-many relationships, MDX can execute all these with simple expressions. The same logic using SQL requires very complex query syntax and some scenarios simply can’t be answered by SQL.
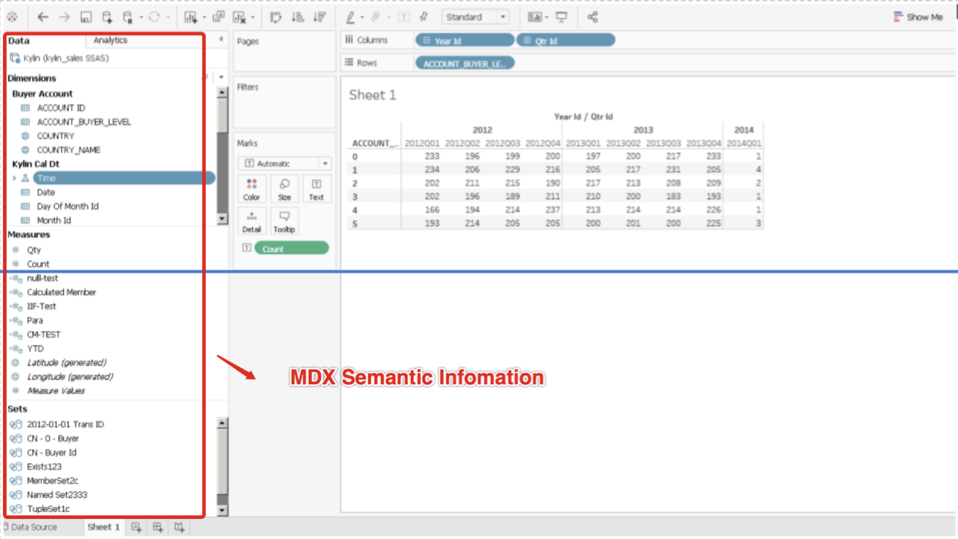
SQL Semantic Model
For the SQL semantic model, only the source table and source columns are included. With SQL, analysts and business users must manually define the model association relationship of the table, the name of the dimension, the aggregation type of the measurement, and the source column order of the hierarchy. Analysis can only be performed after these are defined. This has one advantage, the end-user can flexibly model the data according to the analysis needs, but this also requires the user to have a deep understanding of the underlying data structure.
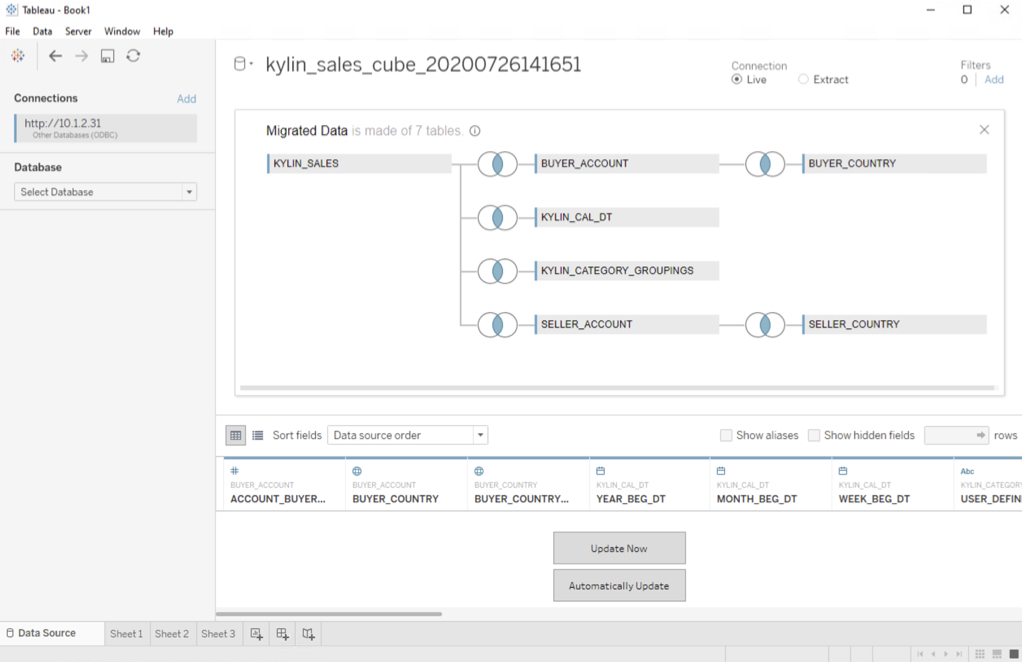
MDX Implementation of Complex Analysis Scenarios
Let's use inventory analysis as an example. Inventory analysis is frequently conducted in the manufacturing, retail, and logistics industries. Among these, the inventory is a semi-additive measurement, meaning it does not have the cumulative property in the time dimension, but has the accumulative property in other dimensions.
Suppose that the inventory record is as reflected in Figure 3 and the total inventory of all products at the beginning (the first day of the month) and the end (the last day of the month) of all products needs to be obtained each month:
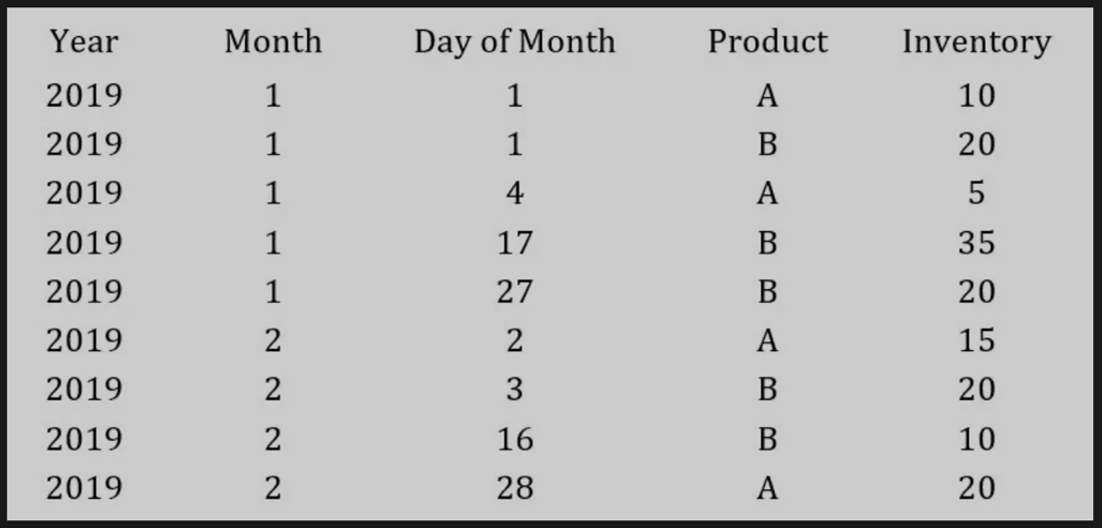
According to our analysis requirements, the results should be as follows:
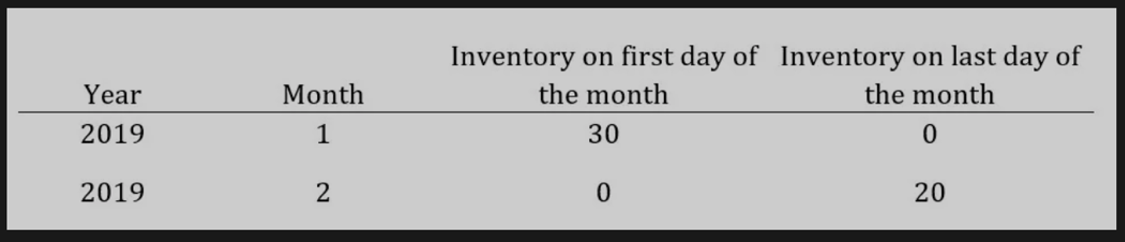
If you use SQL, the query expression is as follows:
- SELECT `year`, `month`,
- SUM (case when `day of month` = 1 then inventory else 0 end) as "Inventory on first day of the month",
- SUM (case when day (last_day(`year` || '-' || `month` || '-' || `day of month`) = `day of month` then inventory else 0 end) as "Inventory on last day of the month"
- FROM inventory group by `year`, `month`
If you use MDX, you need to first define the calculation metric (including the basic metric [Measures].[inventory]=SUM(inventory)), as follows:
- [Measures].[Beginning Inventory] = ([Time].[Month].currentMember.firstChild, [Measures].[Inventory]) [Measures].[End-of-period inventory] = ([Time].[Month].currentMember.lastChild, [Measures].[Inventory])
The MDX query expression is:
- SELECT {[Measures].[opening inventory], [Measures].[End-of-period inventory]} ON Columns,
- [Time].[Month].members ON Rows
- FROM [inventory]
As you can see above, MDX is easier to implement than SQL in inventory analysis scenarios. Similar scenarios include the common account balance analysis in the banking industry and the initial and final value analysis common in the securities industry. In addition, MDX can also support multi-analysis scenarios, which are not supported by SQL.

Kyligence MDX: Enabling Enterprises to Deploy a Unified BI Semantic Layer
Kyligence's AI-augmented analytics platform provides users with standard SQL and MDX interfaces that can seamlessly integrate with all major BI tools. This provides a unified business semantic layer for big data analytics.
Kyligence MDX capabilities give enterprises a way to conduct more complex analysis scenarios that are difficult or impossible with SQL.
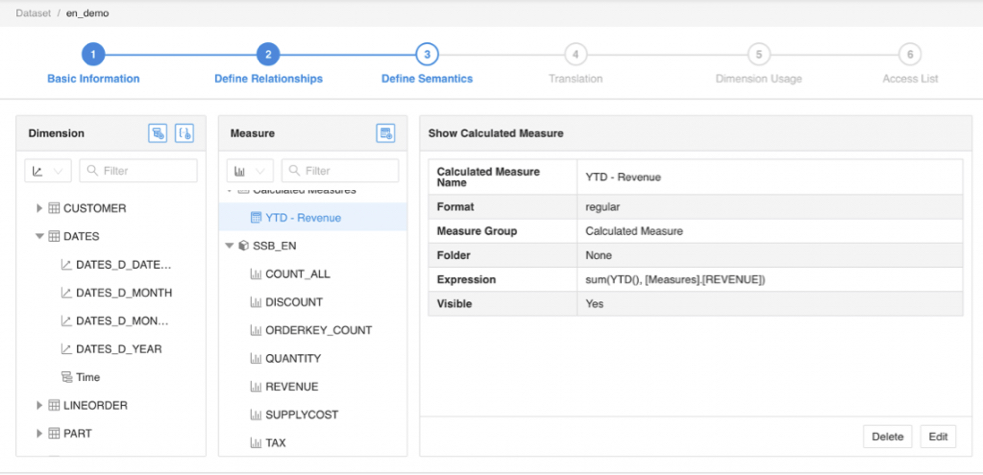
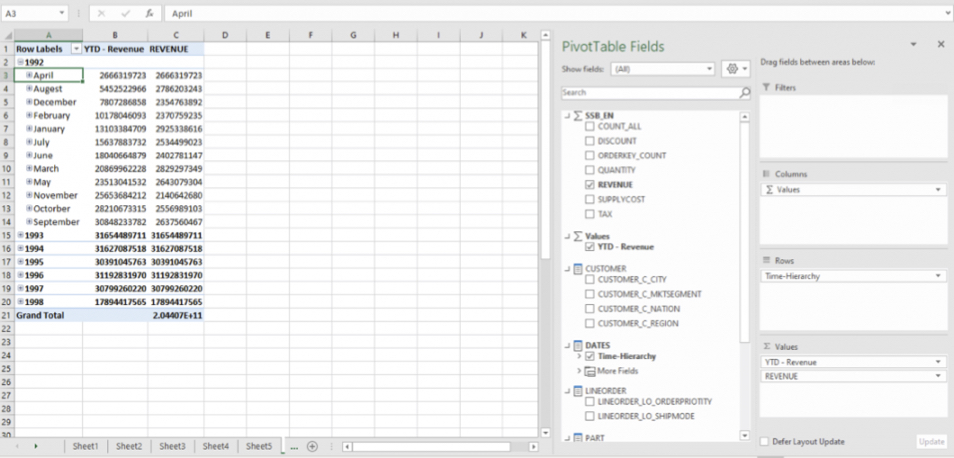
Summary
Both MDX and SQL are frequently used in OLAP queries, and mainstream BI vendors provide support for both interfaces. The difference between the two is:
- MDX queries correspond to multi-dimensional views, while SQL corresponds to relational views. MDX is much simpler in terms of the syntax of aggregate queries.
- The semantic model exposed by the MDX interface is more powerful and business-friendly. The semantic model exposed by the SQL interface is crude by comparison and must be revised and maintained for each BI tool.
- MDX computing and expression capabilities are more abundant and can better support complex analysis scenarios.
In general, if there are complex analysis scenarios in the business such as semi-cumulative, many-to-many, time window analysis, etc. Kyligence MDX is a great solution and can help you deal with those scenarios easily. Your analysts can focus on deriving insights from your business data rather than spending their time struggling with complex data structures and writing complex queries.
TEST DRIVE TODAY with $300 worth of free usage
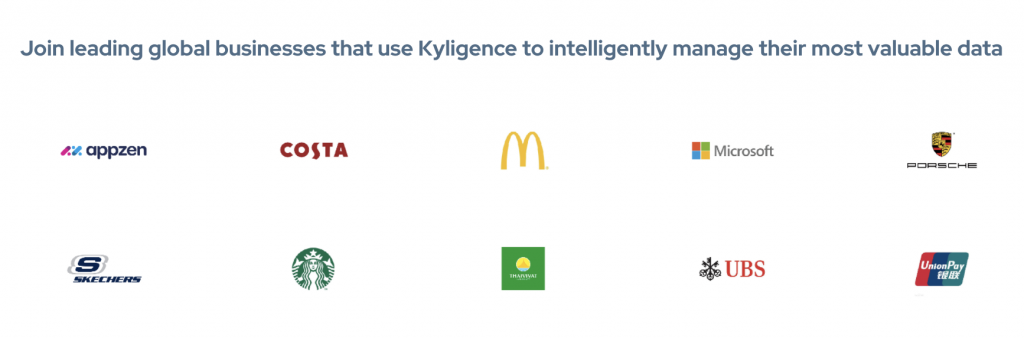